80 % of enterprise AI projects still fail... So what are the keys to success? How can you align artificial intelligence with your strategic objectives? Making a success of an AI project is not something you can improvise: discover the concrete feedback from pioneering companies. A practical look at how to make a success of an AI project.
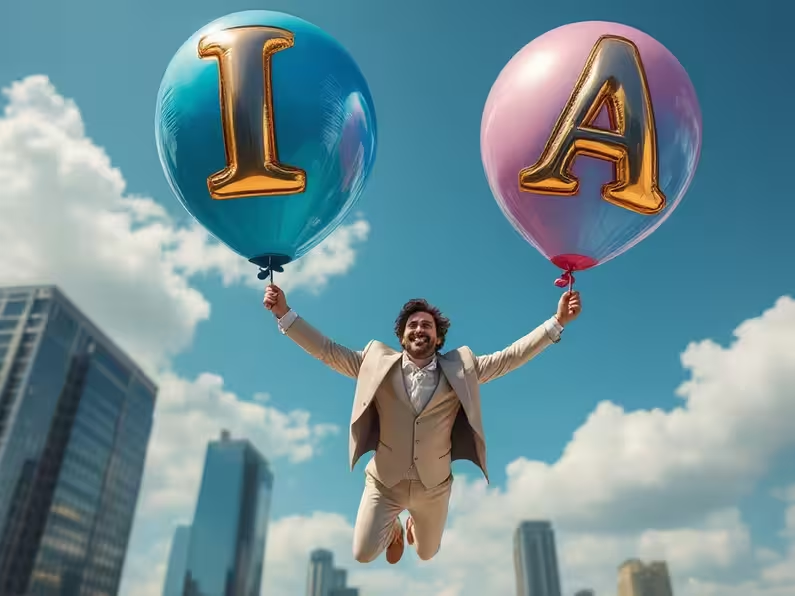
Artificial intelligence promises a revolution: cost optimisation, customer personalisation, disruptive innovation... However, according to various studies (Gartner, RAND), 80 % of AI projects fail before they reach their full potential.
How do you explain this gap between promise and reality? And above all, how can you become one of the 20 successful %s? Using concrete examples from French and international companies, discover the keys to aligning AI with your strategic objectives.
1. AI failures: 8 deadly traps and their solutions
Before exploring the paths to success, it is crucial to understand why so many AI projects get bogged down or fail. Several factors contribute to this high failure rate.
Trap 1 : overestimating the capabilities of AI
In 2024, McDonald's had to abruptly abandon its experiment with voice commands in its drive-throughs and end its three-year collaboration with IBM. The company had overestimated the ability of AI to replace its team members in understanding customer accents and complex requests.
Considering AI as a magic solution that will solve all problems at once, or ignoring its technical limitations, can lead to disappointment and failure.
Solution Include technical experts to assess the feasibility of AI projects and understand the limits of this technology. Adopt a phased approach. Start with pilot projects to test and validate the results before moving on to larger-scale deployments..
Trap 2 Unclear objectives and lack of KPIs
70 % des PoC (Proof of Concept) of AI never go into production. This is often due to a lack of monitoring and performance indicators (KPIs). Many companies embark on AI without clearly defining their objectives or KPIs.
Solution Before taking the plunge, it is crucial to align AI projects with the company's strategic needs. Clearly define the objectives, use cases and KPIs for measuring the success of AI initiatives. Companies should focus on projects that deliver competitive advantage rather than minor improvements. A Point of Delivery (POD) can also helpStarting with 2 or 3 modular units to test feasibility before larger-scale deployment.
Trap 3 Poor quality data
A company like Philip Morris saw a sales analysis project collapse because of incompatible data between different regions.
The effectiveness of AI is highly dependent on the quality of the data used to train the models. Poor quality, incomplete, biased or badly managed data can lead to results that are inaccurate or not in line with expectations.
Solution It is essential to check the completeness, accuracy and relevance of the data. It is also essential to set up processes to standardise, update and eliminate bias data. The data governance and the access management are also key points.
Trap 4 high and unpredictable costs
FedEx is using Salesforce's Agentforce 2.0 platform to streamline its operations, but is facing "terrifying" costs, according to experts quoted by CIO Online magazine, because it autonomous agent uses conversation-based pricing whose return on investment remains unclear. This conversation-based pricing approach forAgentic AI represents a new cost model that is difficult for companies to predict,
According to a RAND report in 2024 , 26 % of AI projects fail due to budgetary problems. The costs associated withGenerative AIThese include infrastructure, model training (GPU) and energy consumption can be high and difficult to predict. Random pricing by suppliers can also complicate project budgeting.
Solutioncompanies should consider usage-based pricing models for better visibility and cost control. It's also a good idea to focus on basic use cases and to develop a clearer understanding of your needs.use SaaS solutions rather than creating bespoke models, particularly for non-technology companies. Optimising energy costs is also an important consideration.
Trap 5: the lack of employee involvement and resistance to change
60 % of employees fear that AI will replace their jobs.
The success of AI also depends on the ability of employees to embrace it. Resistance, disinterest or a disorganised frenzy can compromise the deployment of AI.
Solution It is important to involve employees in the process, to share knowledge, to address fears and to define common objectives. It is essential that our teams are trained in and acculturated to AI. Initiatives such as IA cafés "or AI ambassadors "It is crucial to show how AI can help employees save time and focus on higher value-added tasks. It is crucial to show how AI can help employees save time and focus on higher value-added tasks.
Trap 6 The lack of involvement of the business lines
The Landes MDPH has succeeded in reducing the time taken to process disability files by involving the business teams from the design stage onwards.
AI must not be an isolated initiative of the IT department.
Solution Collaboration between IT and business teams is essential to identify needs, define use cases and guarantee the adoption of solutions.
Trap 7 : lack of monitoring and updating of models
In February 2024, Air Canada was ordered to compensate a customer after a chatbot provided incorrect information on fares. The company tried to absolve itself of responsibility, but the court ruled that it had not taken "reasonable precautions" to check the accuracy of its AI's answers.
AI models require continuous monitoring to ensure that they do not drift and that they remain effective.
Solution : set up test and human validation loops to assess the effectiveness of the models and adjust them on an ongoing basis. Monitor the drift (changes in content) to detect any decline in quality and problems of bias, ethics andhallucination. Société Générale uses human validation loops to reduce the number of false positives 50 % in fraud detection.
Trap 8 : not considering the impact on skills
According to the Global AI Job Barometer 2024 from consultancy and audit firm PwC, 69 % of business leaders worldwide expect AI to require new skills from their employees. What's more, the skills required for occupations exposed to AI are evolving 25 % faster than in jobs less exposed to AI.
AI can automate certain tasks, but it is crucial to invest in retraining and upskilling employees so that they can adapt to the new requirements.
Solution Training: companies should identify future skills needs and put in place training plans to support professional mobility. Decathlon has trained its teams to analyse customer returns, reducing product returns by 15 %.
Success stories: the success of AI projects in companies
Fortunately, some companies have managed to pull themselves up by their bootstraps and have become role models when it comes to AI. Here are a few concrete examples and the lessons we can learn from them.
Company | Sector | Department/Business line | AI use cases | Impact |
---|---|---|---|---|
Société Générale | Bank | Compliance | AI for fraud detection and money laundering | 50% reduction in false positives in fraud detection |
Orange | Telecommunications | Network maintenance | Predictive maintenance network equipment |
|
Carrefour | Distribution | Marketing | Analysis of purchase data to personalise promotional offers |
|
Airbus | Aerospace | Production | Collaborative robots and AI for assembly |
|
Capgemini | Council | HR | AI for recruitment and talent management | 30% reduction in recruitment time |
Decathlon | Distribution | Product design | AI for analysing customer feedback |
|
Atos | IT services | Customer support | AI chatbots for technical support |
|
Bouygues | Construction | Planning | AI for complex project management |
|
These few examples show that AI is not just about increasing productivity. It also helps to improve customer satisfaction and employee well-being (safety, health, reduced mental workload, more rewarding tasks, etc.).
In the final analysis, the adoption of AI and the success of AI projects are not simply a question of technology. It is first and foremost a human adventure, requiring a clear vision, the involvement of all the players and continuous adaptation. By drawing inspiration from success stories and avoiding the pitfalls of failed experiments, you too can make AI a new lever for growth.