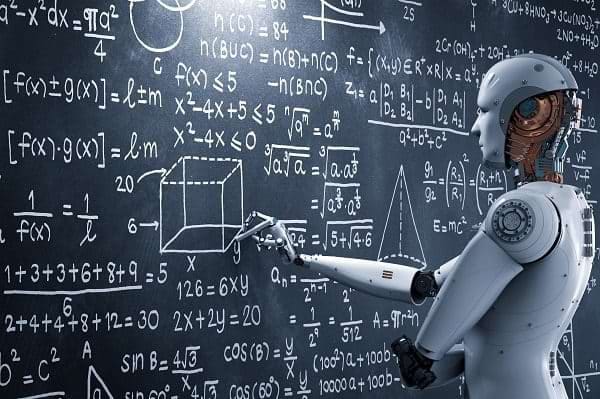
We are living in paradoxical times: after the buzz of recent years, artificial intelligence (AI) is finally a reality for many companies. However, it has to be said that the promises of value creation are often not fulfilled. AI has often been presented as a revolutionary technology that will generate massive new revenues and significantly boost corporate profitability. To explain this gap between rhetoric and reality, we need to understand this technology and the specific features of the projects linked to it.
Artificial intelligence is difficult to define simply. It lies at the crossroads of mathematics, computer science, the social sciences and even philosophy. First of all, let's set aside theSymbolic AIalso known as deterministic. This is the expert systems which led to the development of many major systems in the 70s and 90s.
Today, we generally talk about "probabilistic" AI, which consists of creating value from data using a "probabilistic" approach. statistical approach. This is where we find the flagship concept of the machine learning Algorithms are parameterised with previous data during a learning phase to create a "model" that can be used to build a projection, recognise an image or process language.
Within machine learning, there are several dozen algorithms, each with its own specific features, including the famous deep learning or artificial neural networks. They have remarkable capabilities and performance. They are used in image processing (we'll talk about computer vision) and language (we speak of NLP for Natural Language Processing).
Once these definitions have been established, what are the main applications of AI in business?
Applications in marketing
Marketing is one of the first areas where AI will bring value. In general, this involves using a machine learning approach on previous data to build a model that can be used to segment customers, find out which offer they prefer, predict a potential purchase or personalise the customer experience... and build customer loyalty.
The ability of AI systems to find relevant elements in a mass of data can also make it possible to put in place highly effective marketing targeting approaches (hyper-personalised marketing), or even recommendation systems enabling prospects to be offered only those products likely to be of interest to them.
The performance of this approach is based on the ability of the algorithms to process a large mass of data, but also data of a different nature. In addition to structured customer data (CRM data, web browsing data, etc.), the images and texts (emails, social networks, etc.) used will provide other valuable information about customers. By way of illustration, the use of language processing techniques (NLP) will make it possible to carry out "sentiment analysis" based on written exchanges with the customer in order to automatically determine their level of satisfaction.
Finally, we can't talk about customer relations without mentioning the chatbots. The simplest of these are improved FAQs and do not use AI in the machine learning sense. They offer static scenarios to customers, which has largely contributed to negative user experiences. However, if these scenarios are beefed up with machine learning and NLP, the chatbots will learn over time and will be able to handle "unexpected" exchanges, which will greatly improve the customer experience.
AI in corporate finance
In this field, AI is being used to develop many different types of application, such as income prediction or expenses. Using past data, the algorithm is trained to predict future income based on various current characteristics. Here again, it is interesting to note that in addition to the company's financial data, external data can be used (economic context, weather, etc.) if they can be correlated in some way to the company's activity.
La anomaly detection is one of the other capabilities of certain machine learning algorithms. Very useful in finance, it can be used to detect fraudulent transactions and, more generally, to spot abnormal data that can be used to identify a risk. This is a very useful application for jobs related to quality, compliance, etc. The traditional rules-based approach has been largely superseded by the statistical approach, which allows a large amount of data to be taken into account and, overall, enables risk to be managed more effectively.
AI in industry
Predictive maintenance
AI can be found in predictive maintenance which are widely used in industry. Rather than carrying out maintenance on a system, whatever it may be, every 6 months according to the manufacturer's rule, we will train a machine learning model with past operating data, to have an application capable of indicating the "right moment" to carry out this maintenance or any other modification to the system, which can have a significant financial impact.
Industrial vision for quality
To give another example concerning industrial tools, we can talk about approaches to computer vision to carry out a quality diagnosis. On an industrial production line, an algorithm will be trained on product images to teach it to recognise when there is a defect or a problem... An "intelligent camera" (a camera enhanced by an image processing algorithm) will then be placed on the production line to carry out a visual inspection in the same way as the human eye, only much more efficient!
Automated document processing
Finally, image analysis and language processing technologies are increasingly being used to automate document processing processes that were previously the preserve of humans. These applications, sometimes referred to as intelligent automation, use AI models such as artificial neural networks to automatically recognise and process invoices, process emails or search for information in large volumes of textual data. These advanced technologies replace human beings in repetitive tasks, allowing them to concentrate on higher added-value tasks.
AI ready for use
It is also interesting to note that more and more AI solution providers are offering "off-the-shelf" AI systems, which are ready to use. Companies can integrate these trained models into their business processes relatively simply. This is particularly interesting for SMEs and SMIs with limited data volume and quality, and few in-house expert resources, such as data scientists, capable of building "in-house" solutions.
For example, if it has the right partner, a small business can use solutions on the market to set up a chatbot solution to automate its customer relations, an automatic document processing system, or a computer vision solution to detect faults on its production line, without too much difficulty. In these cases, the ROI (return on investment) can be very rapid!
This non-exhaustive overview illustrates the variety of possible applications for AI, whatever the business sector concerned. Whether it's to automate tasks or to do what human beings are incapable of doing, AI is emerging as the "killer application".
AI project, the obstacles to overcome
However, numerous studies show that, despite sometimes significant investment, most large companies are still finding it difficult to move from small pilot projects to full-scale production, in order to create value.
For their part, smaller companies are still in the process of realising the benefits of AI solutions for them, despite their lower technological maturity.
At this point, it is worth highlighting the main specific features of AI projects that explain some of the difficulties encountered by companies. The first concerns the very concept of machine learning. Very different from a traditional software approach, it can be likened to a system of rules. In machine learning, you don't "programme" the rules of operation or the rules of the game. parameters of the system, they are "learned" via the training data. We are no longer in the world of causality, but in that of correlation and statistics, which implies an iterative design approach, sometimes quite exploratory, with less predictable results.
The second specificity linked to the first is the need for an Agile approach to projects, which is even more collaborative than in traditional IT projects, and which involves data teams as well as business and IT teams, which adds to the complexity.
Finally, preconceived ideas and even fantasies about AI can have a significant impact. For some employees, AI is frightening (our collective unconscious having been fed by decades of dystopian science fiction films), with intelligent robots that could eventually take their jobs. This last, more serious subject has unfortunately been fuelled for years by studies by leading organisations that have been widely reported in the media, and which have proved to be completely false. A famous Oxford study published in 2013 predicted the destruction of 47 % of American jobs by 2023 because of AI!
AI project: what to look out for to succeed
1. Committed managers acculturated to AI
The first is that of the commitment and understanding of the company's managers. We are sometimes surprised to see that senior managers are completely unaware of the opportunity that data represents. It is therefore vital to make them aware of the challenges of AI projects and their specific features, so that they can support AI projects by allocating the right human and financial resources.
By the same token, data and AI literacy is a must for managers, but also for all employees, to encourage the adoption of solutions by business teams.
2. Choose your AI projects wisely
The second subject concerns choosing which projects to launch. In recent years, we have seen dozens of AI pilot projects in large companies. Each business unit had recruited its own team of data science and everyone had a good idea to try out. Good practice, on the other hand, consists of focusing on two or three major subjects, with strong support from senior management and a good level of investment. The choice of the first AI project to be carried out is key, because its success is likely to determine the next stage of investment in AI.
3. Plenty of high-quality, well-managed data
The third topic concerns data and data management. As the saying goes, "no data, no AI". We could add the famous garbage in, garbage out "This means that if the training data for AI models is of poor quality, the result will not be good!
La data managementThis is a long-standing issue for IT departments, and one that is becoming increasingly critical as use cases develop. And while the majority of large companies have put in place genuine data governance (management, access, compliance, quality and data security rules), this remains a major challenge, and one that smaller companies often have to tackle first.
In addition to implementing technical platforms for data management, it is good practice to involve business teams in data governance. This is particularly true when it comes to quality, as data has become a key business "asset" in the same way as industrial plant or human resources.
4. Attracting and retaining talent
The fourth issue is skills. Over the past few years, most large companies have been recruiting data scientistsspecialists in data modelling, as well as data engineers which will manage and prepare the data used in AI.
One of the challenges for these companies will be to retain this talent We are looking for highly sought-after candidates with attractive remuneration packages, autonomy, innovative projects and the development of a genuine data community.
For SMEs, it is not necessarily necessary to recruit this type of profile. Some of these tasks can be carried out by solution publishers and other service providers. For most companies, the main challenge will be to educate the managers and business teams involved in AI projects.
5. Developing skills through training
Faced with a shortage of qualified profiles, companies will be able to use training to develop the skills of their employees, not only in IT but also in other areas (HR, marketing, finance, etc.). The latter can be trained to handle data using simple tools such as Power BI.
In the final analysis, the deployment of AI solutions in companies is not a smooth ride. To create value, management must be committed and employees must be acculturated. What's more, in addition to creating new revenue streams and boosting profitability, AI will in the near future be changing the very nature of work and man-machine relations. It will take over repetitive and codifiable tasks and shake up traditional models of work organisation.
AI could thus enable companies to become more flexible and horizontal, and offer employees the prospect of moving on to higher value-added tasks that are complementary to AI. Certain professions will also disappear and the impact on employment will be significant, even if it is very difficult to make forecasts. All the more reason to be prepared.