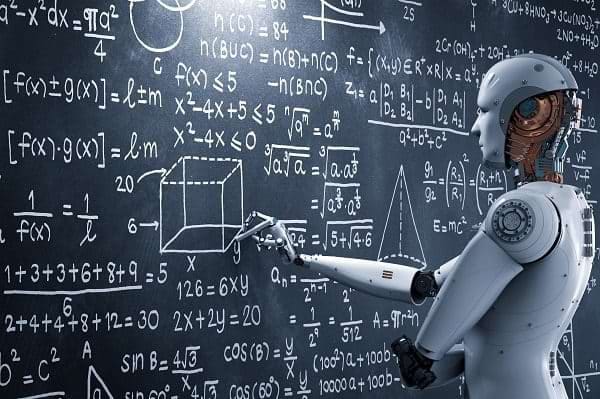
We are living in a paradoxical period: after the buzz of recent years, artificial intelligence (AI) is finally a reality for many companies. However, it is clear that the promises of value creation are often not delivered. However, AI has often been presented as a revolutionary technology that would massively generate new revenues and significantly increase the profitability of companies. To explain this gap between discourse and reality, it is important to understand this technology and the specificities of the projects linked to it.
It’s difficult to simply define artificial intelligence. It is at the crossroads of mathematics, computer science, social sciences, and even philosophy. Let us first put aside theSymbolic AI, also called deterministic. It is that of expert systems which enabled the development of many large systems in the 70s to 90s.
Today, we generally speak of “probabilistic” AI which consists of creating value from data through statistical approach. This is where we find the flagship concept of machine learning : the algorithms are parameterized with previous data during a learning phase to create a “model” which will be used to construct a projection, recognize an image or even process language.
Within machine learning, there are a few dozen algorithms each having specific characteristics, including the famous algorithms of deep learning or artificial neural networks. They have remarkable capabilities and performances. We find them in image processing (we will talk about computer vision) and language (we will talk about NLP for Natural Language Processing).
Once these definitions have been established, in concrete terms, what are the main applications of AI in business?
Applications in marketing
Marketing is one of the first areas where AI will bring value. This generally involves using a machine learning approach on previous data to build a model in order to be able to segment your customers, know which offer they will prefer, predict purchasing potential or even personalize the customer experience... and retain it.
The ability of AI systems to find relevant elements in a mass of data can also make it possible to implement very effective marketing targeting approaches (hyper-personalized marketing), or even recommendation systems making it possible to offer prospects only the products likely to interest them.
The performance of this approach is based on the ability of the algorithms to process a large mass of data, but also data of different natures. Beyond structured customer data (CRM data, Internet browsing data, etc.), the images and texts (emails, social networks, etc.) used will provide other valuable information about customers. For example, the use of language processing techniques (NLP) will make it possible to carry out “sentiment analysis” based on written exchanges with the customer in order to automatically know their level of satisfaction.
Finally, we cannot talk about customer relations without mentioning the chatbots. The simplest of these are improved FAQs and do not involve AI in the sense of machine learning. They provide static scenarios to customers, which has largely contributed to creating negative user experiences. However, if these scenarios are enhanced with machine learning and NLP, chatbots will learn over time and be able to handle “unanticipated” exchanges, which will greatly improve the customer experience.
AI in corporate finance
In this area, AI is used to develop many types of applications such as income prediction or expenses. Using previous data, the algorithm is trained to predict future income based on different current characteristics. Here again, it is interesting to note that beyond the company's financial data, we can use external data (economic context, weather, etc.) if they can be correlated in one way or another to the 'company's activity.
La anomaly detection is one of the other capabilities of some machine learning algorithms. Very useful in finance, it is used to detect fraudulent transactions, and more generally to identify abnormal data which will help identify a risk. A very useful application for professions linked to quality, compliance, etc. The classic approach which is based on rules is largely beaten by the statistical approach which makes it possible to take into account a large number of data and overall to better manage its risk.
AI in industry
Predictive maintenance
We find AI in the systems of predictive maintenance which are widely used in industry. Rather than carrying out maintenance on your system, whatever it may be, every 6 months according to the manufacturer's rule, we will train a machine learning model with past operating data, to have an application capable of indicating the “right time” to do this maintenance or other modification to the system, which can have significant financial impacts.
Industrial vision at the service of quality
To give another example concerning industrial tools, we can talk about approaches to computer vision to carry out a quality diagnosis. On an industrial production line, we will train an algorithm on product images to teach it to recognize when there is a defect, a problem, etc. An “intelligent camera” (camera improved by an image processing algorithm) will be then placed on the production line to carry out a visual inspection like a human eye would do, but much more efficient!
Automated document processing
Finally, image analysis and language processing technologies are increasingly used to automate document processing processes that were previously reserved for humans. Whether to recognize and process invoices automatically, process emails or even search for information in large masses of textual data, these applications, sometimes called intelligent automation, use network-type AI models. of artificial neurons. These advanced technologies replace humans on repetitive tasks to allow them to concentrate on tasks with higher added value.
Ready-to-use AI
It is also interesting to note that more and more AI solution providers are offering “off-the-shelf” AI systems, i.e. ready to use. The company can relatively easily integrate these already trained models into its business processes. This is very interesting, in particular for SMEs and ETIs which have limited data in volume and quality, as well as few internal expert resources, such as data scientists, capable of building “in-house” solutions.
Thus, a small business will be able to use market solutions to set up, without too much difficulty, if it has the right partner, a chatbot solution to automate its customer relations, an automatic document processing system, or even a solution of computer vision to detect defects on its production line. In these cases, the ROI (return on investment) can be very rapid!
This non-exhaustive overview illustrates the variety of possible applications of AI, whatever the sector of the company concerned. Whether to automate tasks or to do what humans are unable to do, AI appears to be the “killer application”.
AI project, the obstacles to overcome
However, numerous studies show that, despite sometimes significant investments, most large companies still have difficulty moving from small pilot projects to full-scale production, in order to create value.
For their part, smaller companies are still realizing the benefits of AI solutions for them, despite lower technological maturity.
At this stage, it is interesting to highlight the main specificities of AI projects which explain part of the difficulties encountered by companies. The first concerns the very concept of machine learning. Very different from a classic software approach, it can be compared to a system of rules. In machine learning we do not “program” the operating rules or parameters of the system, they are “learned” via the learning data. We are no longer in the world of causality, but in that of correlation and statistics, which implies an iterative design approach, sometimes quite exploratory, with less predictable results.
Second specificity linked to the first: the need to have an Agile approach to projects, even more collaborative than in traditional IT projects, and which involves, in addition to business and IT teams, data teams, which adds complexity.
Finally, it is the preconceived ideas, even fantasies concerning AI, which can have a significant impact. For some employees, AI is scary (our collective unconscious having been fueled by decades of dystopian science fiction films), with intelligent robots that could eventually take over their jobs. This last, more serious subject was unfortunately fueled for years by studies by reference organizations which were widely reported in the media, and which turned out to be completely false. A famous Oxford study published in 2013 predicted the destruction of 47 % American jobs by 2023 because of AI!
AI project: points to watch out for to succeed
1. Leaders engaged and acculturated to AI
The first is that of the commitment and understanding of the company's leaders. We are sometimes surprised to see that leaders completely do not realize the opportunity that data represents. It is therefore essential to make them aware of the challenges of AI projects and their specificities so that they support AI projects by allocating the right human and financial resources.
Relatedly, acculturation to data and AI is a must for managers, but also for all employees, to promote the adoption of solutions by business teams.
2. Choose your AI projects wisely
The second subject concerns the choice of projects to launch. In recent years, we have seen too many dozens of pilot projects implementing AI within large companies. Each business unit had recruited its own team of data science and everyone had a good idea to try. On the contrary, good practice consists of focusing on two or three major subjects, with strong support from management and a good level of investment. The choice of the first AI project carried out is key, because its success risks conditioning subsequent investments in AI.
3. Data in quantity, quality and well managed
The third topic concerns data and its management. As the saying goes, “no data, no AI”. We could add the famous “ garbage in, garbage out » which means that if the training data of the AI models is of poor quality, the result will not be good!
La Data managment, an old subject for IT departments, becomes critical as use cases develop. And if large companies have for the most part implemented real data governance strategies (rules for management, access, compliance, quality, data security), this remains a major issue and often a subject to initiate for smaller companies.
Beyond the implementation of technical data management platforms, a good practice consists of involve business teams in data governance. This is particularly relevant to the issue of quality, because data has become key business “assets”, in the same way as an industrial tool or human resources.
4. Attract and retain talent
The fourth topic is skills. Most large companies have, in recent years, recruited data scientists, specialists in data modeling, but also data engineers who will manage and prepare the data used in AI.
One of the challenges for these companies will be to retain these talents highly sought after through an attractive remuneration policy, but also autonomy, innovative projects and the development of a real data community.
For mid-sized companies and SMEs, it is not necessarily necessary to recruit this type of profile. These tasks may be carried out in part by solution publishers and other service providers. For most companies, the main issue remains the acculturation of managers and business teams involved in AI projects.
5. Develop skills through training
Faced with a lack of qualified profiles, companies will be able to use training to develop the skills of their employees, those in IT of course, but also those in the professions (HR, marketing, finance, etc.). They will be able to train in data manipulation using simple tools like Power BI.
Ultimately, deploying AI solutions in business is not a smooth ride. Value creation requires management commitment and employee acculturation. Furthermore, beyond the creation of new revenues and increased profitability, AI in the near future will change the very nature of work and human-machine relationships. It will take care of repetitive and codifiable tasks and will shake up traditional models of work organization.
AI could thus allow companies to become more flexible, more horizontal and offer employees prospects for advancement towards tasks with higher added value and complementary to AI. Certain professions will also disappear and the impact on employment will be significant, even if it is very difficult to make predictions. All the more reason to prepare.