A bias in artificial intelligence (AI) refers to the tendency of an algorithm to produce biased results or decisions, favouring or disfavouring certain groups or individuals.
It reflects human biases or structural flaws in data, learning methods or the design of AI models. These biases can lead to unfair, discriminatory or inaccurate decisions, affecting specific groups or individuals disproportionately.
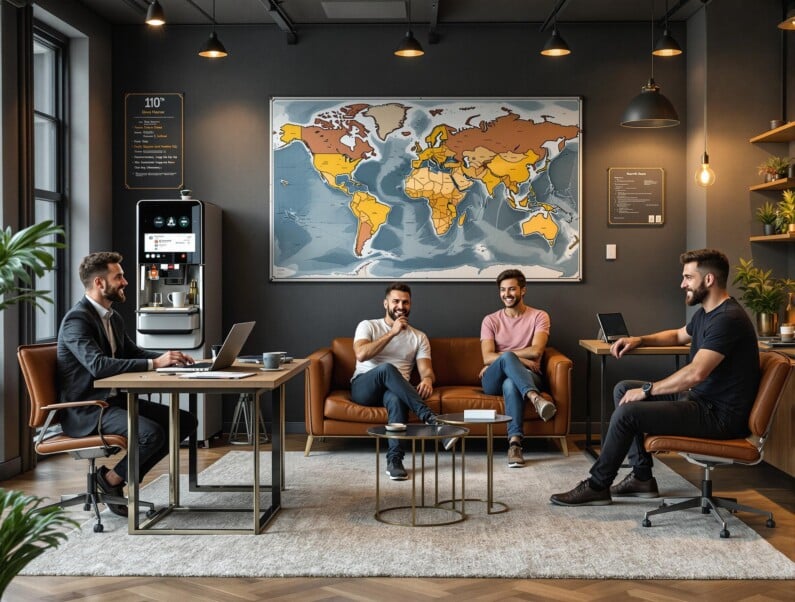
Example of AI bias:
When you ask an AI to generate an image with managers, the AI creates an image showing only white, young, handsome, bearded men with hair and no glasses...
©Alexandre SALQUE / ORSYS le mag
Origins of bias in AI
- Non-representative training data
- For example: datasets dominated by white males cause facial recognition systems to identify women or dark-skinned people less well.
- Case in point: Amazon's recruitment tool, based on historically male CVs, systematically penalised female candidates.
- Algorithmic biases
- Unsuitable choice of variables or metrics (e.g. optimising overall accuracy at the expense of equity between demographic groups).
- Example: bank lending algorithms that use indirect criteria (neighbourhood) to discriminate on racial grounds.
- Cognitive biases of developers
- The unconscious prejudices of data scientists (e.g. associating certain jobs with a particular gender) are reflected in the models.
- Confirmation bias
- AI reinforces existing stereotypes by relying on historically biased data (e.g. gendered translation in Google Translate).
👉 Common types of bias
-
Selection bias Non-representative data. (e.g. under-represented minorities, limited geographical data). Training data not reflecting the overall reality.
-
Measurement bias incorrect/incomplete data. (e.g. labelling errors, partial medical data). Problems with data collection or labelling.
-
Exclusion bias Omission of key variables. (e.g. ignoring school history for success). Important factors neglected in the model.
-
Stereotypical biases Reinforcement of clichés. (e.g. "CEO" = white man). Reproduction of societal stereotypes by AI.
-
Aggregation bias : masking differences. (e.g.: average income masking inequalities). Combination of data erasing significant variations.
-
Confirmation bias confirming prejudices. (e.g. web research favouring developers' theories). AI validating pre-existing ideas.
-
Anchoring bias overestimation of the first information received. (e.g. property price overly influenced by initial price). Overemphasis on the first piece of information.
-
Attribution bias wrong cause and effect. (e.g. fraud linked to a region, not to the individual, incorrect medical diagnosis based on a superficial correlation between two symptoms). Incorrect causal link.
-
Presentation bias Influence through display. (e.g. biased "recommendations" in e-commerce). Results presented in a biased way.
-
Historical bias Reproduction of the biased past (e.g. recruitment reproducing under-representation of women). Learning and perpetuating historical biases.
-
Interaction bias user interaction bias. (e.g. : chatbot biased by complaints from certain groups). User interaction modifying AI behaviour.
-
Valuation bias Biased evaluation of performance. (e.g. unfair facial recognition test). Non-objective measurement of model performance.
💥 Consequences
The consequences of bias in AI can be serious and affect many areas:
- Discrimination and injustice : biased AI systems can perpetuate and even amplify existing discrimination against certain groups (e.g. in employment, credit, criminal justice, health, etc.).
- Incorrect or ineffective decisions : a biased AI system can make incorrect or less effective decisions, because it is based on a distorted representation of reality.
- Loss of confidence : Bias can erode public confidence in AI and the technologies that stem from it.
- Ethical issues : The bias raises important ethical questions about the fairness, equity, accountability and transparency of AI systems.
💉 Solutions to reduce bias
- Data diversification
- Enrich training games with a variety of samples (e.g. add faces of all ethnicities for facial recognition).
- Audits and A/B tests
- Compare AI performance on different demographic groups before deployment.
- Algorithmic transparency
- Use tools such as AI Fairness 360 (IBM) or What-if Tool (Google) to detect bias.
- Multidisciplinary teams
- Involve experts in ethics, sociology and law to counterbalance technical bias